Share to gain more social capita
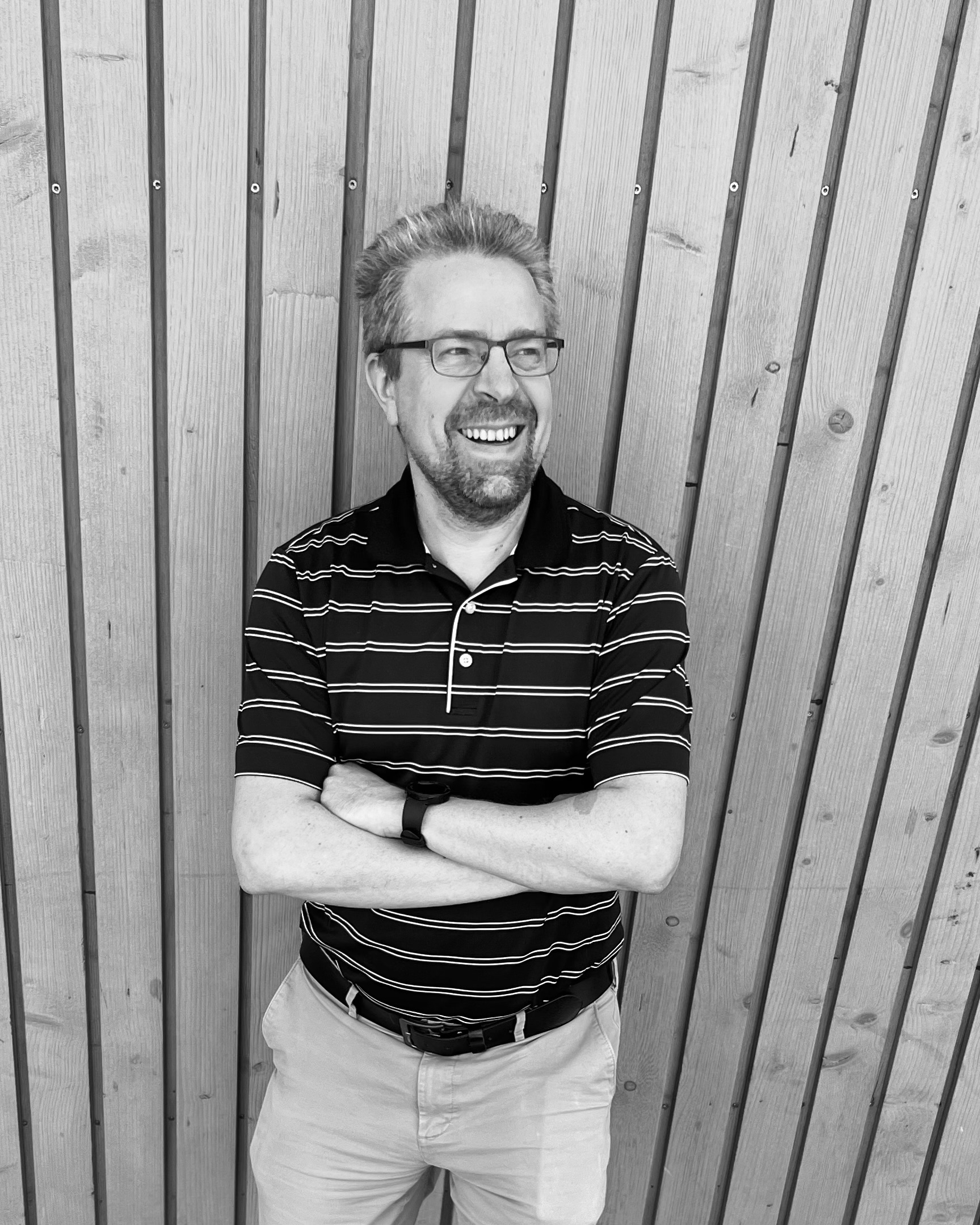
Written by — Sami Helin, Data Architect
Data catalogs are a powerful tool that can help companies effectively manage their data assets. Our Data Architect Sami sheds light on the tool and helps you discover how it can be the missing piece in your data management toolbox.
Written by — Sami Helin, Data Architect
Share to gain more social capita
The evolution of the data catalog market has been rapid in recent years. It has come a long way from data development-oriented metadata management systems and typically non-existing business-friendly documentation. For those of you who are new to data catalogs, in short, the main purpose is to help users find and trust the data.
It seems data catalogs have become a more popular data topic, but many organizations haven’t yet deployed one. There are also a lot of obscurities related to data catalogs; e.g. what is the purpose, is there a lot of manual work, and what should they contain?
This blog series tries to give some insights into the data catalog market, use cases, and practical tips on useful content. There are two parts: the first part will focus on understanding data catalog purpose and main drivers within your organization. The second part goes deeper into possible content areas in a data catalog to give some practical tips on how to get started.
As already mentioned, the market has developed a lot. Earlier, the data catalog market was mostly identified by Enterprise catalogs (Alation, Collibra to name a few) which offer broad functionality, but are also quite maintenance heavy and expensive.
Cloud ecosystems provide their own tooling to keep track of the data, too. Different tools within the data stack (e.g. data transformation tool, reporting tool) also carry functionality to further describe the data and work as a lightweight data catalog. Of course, the limits in these approaches come somewhat from the technical boundaries – be it then the specific ecosystem or tool, it is not as much directed to evolving into more diverse solutions as data catalog generalists. Typically the approach is also directed to technical tool functionalities and main stakeholders of the technical stack rather than the actual business end users.
More recent evolution has happened regarding SaaS data catalogs. A growing number of data catalogs are provided with a monthly fee with low setup and maintenance effort when enterprise catalogs typically are quite resourcing and budget heavy. End-to-end visibility to data and describing organizations’ business data environment has come to reach for most companies.
With the ease of setting up and lower cost of these newer approaches, it is starting to become questionable why data catalog shouldn’t be part of the basic data stack next to e.g. data pipeline, data storage, and data utilization tools.
A data catalog is obviously only a tool: You need to know why you need it and what you need it for. But the impression that data cataloging is heavy on manual maintenance and an expensive initiative is no longer valid.
So why do you need a data catalog? This requires thinking of the key stakeholders and requirements in your organization, but there are several resources to give ideas in this area. Different vendors have their own lists (highlighting the importance of their strengths, of course), but data catalogs have been a popular subject in more objective sources of data governance and data management as well; for example, Dataversity and techtarget.com can act as good first steps.
In general, the main focus in data catalogs is at the moment typically on data discoverability and different dimensions in understanding the data (where it comes from, what are business understandable descriptions etc.). The main user group is people trying to find relevant data for their use cases – often an analyst type of user. In many cases, this has led to database descriptions with a limited understanding of the business point of view. Alignment to business terminology and concepts needs to be ensured.
For broader data governance and business alignment, it is useful to describe e.g. business use cases, data stakeholders, and processes related to data. In other words, why do we have the data, and who is using it for which purposes? That would enable a better connection of data to business operations and value.
From a data operations point of view, the status and observability of the data bring actual daily data status and managing data issues into catalogs. Data development linked to data catalogs facilitates data initiatives and backlog management.
There are many directions within data management that can be supported by effectively managing metadata and describing data within organizations. It remains to be seen which functionality data catalogs will evolve to cover and how the tooling will converge.
Regardless of the future direction, thinking about what is useful to describe from your data and who utilizes those descriptions will pay off. Implementation itself can be automated from a growing set of data stack components and the understanding you describe about your business related to data can in general be transferred to your preferred tooling. Of course, some are better fit depending on your use case, but rather than looking for the ultimate solution, more focus should be on the content and the stakeholders than the tooling itself.
It is also relatively safe to choose an initial best-fit approach for a data catalog. Switching to another tool as requirements evolve shouldn’t be a huge effort after all. Technical structures used to harvest system descriptions follow similar principles regardless of the tool and importing and exporting descriptions is typically an out-of-the-box functionality. More important is to consider what is useful to describe and why. And start that work to enhance your understanding.
Depending on the objectives and use cases, it is good to think through your specific needs and requirements. Of course, it is also good to choose tooling that fits your technological environment to ensure needed automatic description updates. There is a wide number of catalogs supporting the typical use cases and taking your data understanding and documentation to the next level.
And for those of you waiting for ChatGPT or similar AI models to overcome the need for a data catalog, there is still a need to feed those models with quality metadata. And as such, the thinking of relevant data describing content is a future-proof path ;-)
Share to gain more social capita
Written by — Sami Helin, Data Architect